Machine learning in cancer-associated thrombosis: hype or hope in untangling the clot
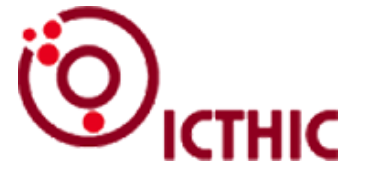
Accepted: 22 March 2024
All claims expressed in this article are solely those of the authors and do not necessarily represent those of their affiliated organizations, or those of the publisher, the editors and the reviewers. Any product that may be evaluated in this article or claim that may be made by its manufacturer is not guaranteed or endorsed by the publisher.
Authors
The goal of machine learning (ML) is to create informative signals and useful tasks by leveraging large datasets to derive computational algorithms. ML has the potential to revolutionize the healthcare industry by boosting productivity, enhancing safe and effective patient care, and lightening the load on clinicians. In addition to gaining mechanistic insights into cancer-associated thrombosis (CAT), ML can be used to improve patient outcomes, streamline healthcare delivery, and spur innovation. Our review paper delves into the present and potential applications of this cutting-edge technology, encompassing three areas: i) computer vision-assisted diagnosis of thromboembolism from radiology data; ii) case detection from electronic health records using natural language processing; iii) algorithms for CAT prediction and risk stratification. The availability of large, well-annotated, high-quality datasets, overfitting, limited generalizability, the risk of propagating inherent bias, and a lack of transparency among patients and clinicians are among the challenges that must be overcome in order to effectively develop ML in the health sector. To guarantee that this powerful instrument can be utilized to maximize innovation in CAT, clinicians can collaborate with stakeholders such as computer scientists, regulatory bodies, and patient groups.
How to Cite

This work is licensed under a Creative Commons Attribution-NonCommercial 4.0 International License.
PAGEPress has chosen to apply the Creative Commons Attribution NonCommercial 4.0 International License (CC BY-NC 4.0) to all manuscripts to be published.
Most read articles by the same author(s)
- Maria J. Fernandez Turizo, Rushad Patell, Jeffrey I. Zwicker, Identifying novel biomarkers using proteomics to predict cancer-associated thrombosis , Bleeding, Thrombosis and Vascular Biology: Vol. 3 No. s1 (2024): 12th ICTHIC
Similar Articles
- Cristina Santoro, Alessandro Casini, Addressing some challenges of congenital fibrinogen disorders in 2023 and beyond , Bleeding, Thrombosis and Vascular Biology: Vol. 2 No. 3 (2023)
- Pier Mannuccio Mannucci, Growing weapons to fight hemophilia , Bleeding, Thrombosis and Vascular Biology: Vol. 2 No. 1 (2023)
- Luca Puccetti, Vincenzo Sammartano, Federico Caroni, Margherita Malchiodi, Paola Calzoni, Eleonora Franceschini, Lucrezia Galasso, Monica Bocchia, Safety of COVID-19 mRNA vaccination in patients with history of acquired hemophilia A: a case series , Bleeding, Thrombosis and Vascular Biology: Vol. 1 No. 3 (2022)
- Pier Mannuccio Mannucci, Gene transfer in hemophilia A: not cogent yet , Bleeding, Thrombosis and Vascular Biology: Vol. 1 No. 1 (2022)
- Stefania Momi, Paolo Gresele, Blood platelets and Charles Darwin’s natural selection , Bleeding, Thrombosis and Vascular Biology: Vol. 2 No. 1 (2023)
You may also start an advanced similarity search for this article.